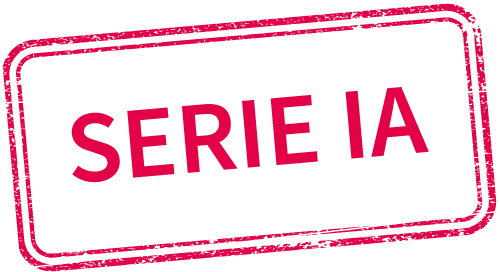
Customer relations
Deploying banking chatbots
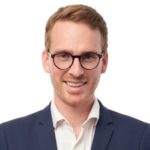
Alexandre Jotterand
(Translated by DeepL)
Recent advances in generative artificial intelligence (AI) have rekindled financial players’ interest in chatbots for managing customer relationships. According to a report by the US Consumer Financial Protection Bureau (CFPB) published in 2023, around 37 % of Americans interacted with a banking chatbot in 2022, and all the major US banks are using them.
However, their deployment raises many questions. Some of these are not new, particularly concerning the management of data and subcontractors ; others are more specific to the technology used : automated decisions, risks of error, discrimination, and so on. In this contribution, we will focus on this second category of issues, and more specifically on aspects relating to the control of chatbot responses and the consequences of errors.
Differences between deterministic and statistical systems
It is now well known that AI systems can make mistakes and hallucinate. But it is worth remembering that this has not always been the case : previous generations of chatbots were based on knowledge bases generated by experts (deterministic algorithmic systems). These deterministic systems provide predictable answers, but are sometimes not very useful.
The new generations of chatbots use generative AI models, based on Large Language Models (LLM). Because of their statistical nature, these systems can be unpredictable and provide factually incorrect or biased responses, posing risks for the banks that deploy them. Indeed, in its report mentioned above, the CFPB is highly critical of their use, concluding that “the use of conversational chatbots trained on LLMs in banking [is] an unreliable source for responding to customers“.
Contractual aspects
This characteristic raises the question of contractual risks in the event of the provision of inaccurate information resulting in financial damage. In Moffatt v. Air Canada, 2024 BCCRT 149, a Canadian court ruled for the first time to our knowledge that a company is liable for information provided by its chatbot, in the same way as for all ‘static’ information on its website. The court held that the company had not taken the measures reasonably necessary to ensure that its chatbot provided correct information.
Transposed to the banking sector in Switzerland, this case would, in our view, raise the issue of liability exclusions, which would be stipulated in the general terms and conditions of the service. Such exclusions will only be valid to the extent permitted by law, in particular art. 100 of the Swiss Code of Obligations or the rules on unfair competition (in particular the rules on unusual clauses). The banks’ room for manoeuvre will be reduced, since the limitations set out in art. 100 para. 2 CO for licensed industries will in principle apply to them. For example, a judge could hold null and void a clause that excludes slight negligence on the part of the bank. Under art. 101 para. 3 of the Swiss Code of Obligations, however, the bank will be able to exclude minor negligence on the part of its auxiliary. The question then arises as to whether the third-party service providers involved in the process of making the chatbot available are auxiliaries or not. Given the potential number of players involved alongside the bank (supplier of the LLM, databases, or AI system, developer, integrator, or operator, etc.) and the complexity of the relationships, this question will require a concrete analysis of the roles and responsibilities of each.
Regulatory aspects and recommendations
FINMA has not issued a specific opinion on chatbots. In its “Risk Monitoring 2023“, it adopted a pragmatic and open position on the use of AI. Like the processes they replace, AI systems must comply with all applicable laws, as well as with existing FINMA circulars. Nevertheless, FINMA identifies four areas of specific attention for AI : “governance and accountability”, “robustness and reliability”, “transparency and explainability”, and ‘equal treatment’.
FINMA does not spell out how these four areas are to be addressed in concrete terms. Fortunately, a number of frameworks exist for establishingtrustworthy AI management, such as the OECD’s “Principles on AI” or the NIST’s “AI Risk Management Framework“.
Generally speaking, the deployment of AI must be integrated into multidisciplinary governance and follow a clear strategy to ensure that the technological solution envisaged is both necessary and capable of meeting the identified need. Robustness and explainability will then be particularly critical for chatbots based on LLMs. There are various technical measures for improving these aspects – for example, concerning the choice of model and training algorithms, RAG [Retrieval Augmented Generation] and fine tuning techniques, as well as testing and validation – which will need to be implemented throughout the lifecycle of the AI system. We would stress here the importance of monitoring the system during deployment, which we believe will be an important element in assessing regulatory compliance and liability (particularly in terms of fault).
Finally, care must be taken to ensure that users are properly informed about the use of AI and the capabilities and limitations of chatbots, and that appropriate conditions of use for services are drawn up.